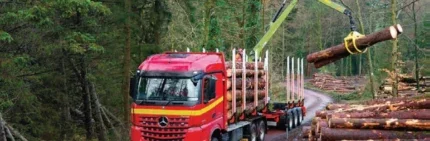
The logistics industry, encompassing transportation, warehousing, and distribution, is undergoing a significant transformation driven mainly by artificial intelligence (AI).
Transport and logistics have traditionally adopted planning methods like load optimisation, route planning, scheduling and warehouse operations, often achieved through mixed-integer linear programming (MILP) techniques. However, these methods, though well-proven, are limited by their inability to handle the real-world complex, non-linear nature of transport and logistics. Enter AI-driven optimisation, which has begun to revolutionise the industry by offering dynamic, real-time solutions that can adapt to changing conditions, optimise resources more effectively, and ultimately enhance efficiency, compliance, and customer satisfaction.
TRADITIONAL METHODS: LIMITATIONS AND CHALLENGES
In the transport and logistics sector, traditional optimisation methods, including day-ahead or week-ahead planning for transport operations, have relied heavily on mathematical methods like linear programming. These models, such as MILP, are effective for load planning, routing and scheduling tasks. In warehouse operations, a typical application is slotting. This process involves positioning stock-keeping units (SKUs) in locations that minimise pick-path distances.
Despite the advantages, MILP faces several inherent limitations. The primary challenge is that the real world is not linear – routes, customer demands, and regulations create complex relationships. Moreover, MILP struggles with highly complex regulations and non-linear constraints. As a result, solving these models can take an unacceptably long time, and the outcomes may not always reflect the dynamic and multi-faceted nature of real-world logistics challenges.
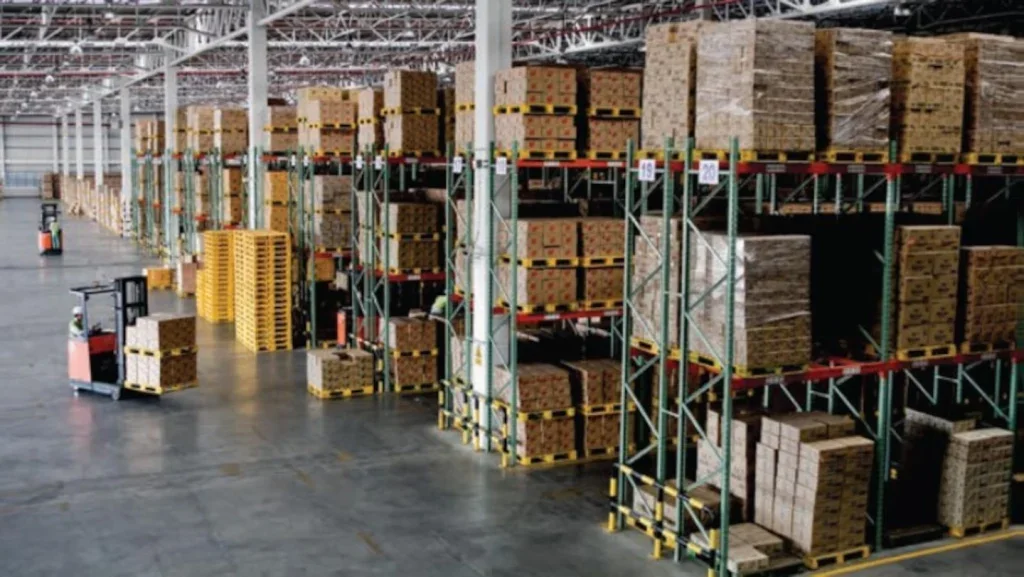
For example, consider a company that needs to dispatch vehicles daily based on fluctuating customer orders. Traditional MILP methods can optimise this dispatch beforehand but fall short when unexpected delays or order changes arise during the day. Additionally, while MILP models work relatively well in tactical planning scenarios where a perfect plan isn’t essential, they fall short in real-time applications. This situation creates a major problem: plans generated by MILP often require further interpretation and manual adjustment by human planners, adding complexity rather than simplifying the process. Thus, the adoption of these systems has remained patchy, with many companies wary of the increased workload without a clear improvement in operational efficiency.
EMERGENCE OF AI DRIVEN OPTIMISATION
AI-driven optimisation presents a fundamentally different approach. Companies like Opturion have pioneered AI technologies tailored to logistics, focusing on compliance, customer service, and cost efficiency. These AI systems process complex datasets to produce compliant solutions that ensure customer service standards are met while continuing to seek cost reductions in real time. Crucially, these systems do not require simplified problem models and can accommodate highly complex rules and constraints.
AI optimisation provides a critical edge over traditional methods due to its ability to handle real-time changes effectively. Once an initial solution is found, the algorithm searches for better solutions within a predetermined timeframe, constantly refining its results to lower costs without compromising compliance or service quality. This process, driven by advanced machine learning algorithms, allows for a rapid re-optimisation, ensuring that the logistical plans remain dynamic and adaptable throughout the day.
Furthermore, AI-powered systems can address many complexities that MILP and other traditional methods cannot. For example, warehouse slotting has traditionally been addressed in simplistic ways, such as positioning fast-moving items near the loading dock to minimise retrieval times. However, orders often include slow-moving items in industries like spare parts or grocery delivery, which complicates the optimisation. AI-driven slotting optimises the entire picking process by modelling it over extended periods, such as a month or a year of historical pick data. The AI algorithm considers picking distance but also variables like stackability, fragility, and customer order distributions, ultimately reducing inefficiencies in the warehouse. Similar applications arise in the transport sector, such as decisions about fleet composition and warehouse location. Any potential solution must be validated across various scenarios, from low seasonal demand to peak periods. This exercise can also include comparing the cost of owned vehicles and contractors.
Two of the critical challenges in transport and logistics are variability and uncertainty. Variability, for example, in customer orders or volumes, significantly impacts the efficiency of assets with fixed capacity, like transport fleets and distribution centres, and any decision must take full account of the underlying complexities. AI can solve such complex problems without simplification, leading to robust decision-making and good economic outcomes. Uncertainty, such as short-term changes in customer requirements or the unavailability of assets and staff, must also be managed. The fast execution of AI enables the organisation to react and recover most efficiently,
TRANSFORMATIONAL IMPACTS
AI-driven optimisation has delivered transformational results across various logistics applications. Here are several key areas where AI has significantly improved logistics operations:
- Dispatch optimisation: AI has transformed dispatch operations for courier services, taxi trucks, and non-emergency patient transport. Each new job can be assigned dynamically, with the AI system adjusting vehicle routes in real time. The system considers job suitability, vehicle availability, and the impact on already scheduled jobs. This results in greater punctuality – improving on-time performance by up to 25% – and enhances efficiency by as much as 10%. Additionally, these systems are highly scalable; a single server can manage over 1,000 vehicles, delivering new optimised solutions every minute.
- Automating back-office operations: One of the most exciting outcomes of AIdriven optimisation is its potential to automate back-office operations entirely. AI can communicate directly with drivers, reducing the need for human dispatchers. This decreases the chances of human error and significantly reduces operational workload. The rapid and detailed nature of AI-generated plans ensures that transport and logistics companies can rely on automated systems without requiring constant human oversight.
- Replacing legacy systems: Many logistics companies still rely on legacy optimisation systems, often producing only approximate plans. AI-powered systems are faster and far more accurate, offering detailed plans that allow for precise management of drivers and contractors. Companies that have transitioned from legacy systems to AI-driven platforms have reported more than 15% savings through increased vehicle utilisation and more efficient routing and scheduling.
- Supply chain optimisation: Traditional supply chain optimisation, particularly in industries like bulk chemicals or manufacturing, has been limited in scope due to the complexity of the problem. These systems often rely on simplified models, such as aggregating production in weekly or bi-weekly buckets, which may work for specific industries but are ineffective for sectors where every day brings significant changes. AI, however, can model production, transport, and logistics activities in granular detail, producing comprehensive plans that improve coordination across the supply chain.
- Strategic optimisation: AI has a key role to play in more strategic decisions, such as the location of facilities, fleet size and composition. Typically these questions are modelled in spreadsheets with many simplifications. AI optimisation ensures the decision is robust against all potential scenarios and provides rich information on occupancy and utilisation over the extended planning period.
THE FUTURE OF AI IN LOGISTICS
As AI-driven optimisation evolves, its impact on logistics will only grow. The ability of AI systems to process vast amounts of data, adapt to real-time changes, and continuously optimise operations presents immense opportunities for logistics companies to reduce costs, improve service quality, and streamline operations.
Moreover, the flexibility of AI allows it to be deployed across various sub-sectors within logistics, from road transport and warehousing to more specialised areas like supply chain optimisation for advanced manufacturing. By embracing AI-driven technologies, logistics companies can stay ahead of the competition, delivering more efficient and reliable customer services.
In conclusion, AI-driven optimisation is set to revolutionise logistics in the coming years. By overcoming the limitations of traditional optimisation methods and enabling real-time, dynamic planning, AI is transforming how transport and logistics companies manage their operations. From dispatch optimisation and warehouse slotting to automating backoffice processes, AI offers a more intelligent, faster, and more efficient way to manage the complexities of logistics, paving the way for a future where human oversight is minimal, and operations are streamlined and optimised to perfection.